AI in Traditional Industries: Practical Steps to Embrace the Future
Adopting artificial intelligence (AI) in traditional industries like manufacturing, logistics, and healthcare can seem overwhelming. However, with thoughtful strategies and relatable examples, AI can become a valuable tool rather than a disruptive force. In this guide, we’ll explore the common challenges, practical solutions, and inspiring success stories that demonstrate AI’s transformative potential.
Why AI Adoption Feels Intimidating in Traditional Industries
Cultural resistance is a significant barrier to AI adoption in traditional industries. Many companies have relied on the same processes for decades, creating a natural resistance to change. Employees often fear that AI will replace their jobs, which can exacerbate this hesitation. To counter this, companies should focus on transparency and framing AI as a tool that complements human work rather than replacing it.
Another challenge is the skills gap. Many workers in industries like manufacturing and logistics are not trained in technology. Terms like “machine learning” or “neural networks” can sound intimidating. Organizations can address this by offering accessible training programs through platforms like Coursera, LinkedIn Learning, or DataCamp, which provide tailored courses for non-technical users.
Legacy systems pose another hurdle. Older IT systems in factories or hospitals often cannot integrate seamlessly with modern AI solutions. Upgrading these systems incrementally, rather than all at once, can make the transition manageable.
Finally, there’s the uncertainty around return on investment (ROI). Companies fear the costs of implementing AI without a guarantee of value. Highlighting case studies of success, such as predictive maintenance that reduces downtime and costs, can help mitigate these concerns.
Making AI Relatable: Changing Perceptions
One way to ease the transition is by using analogies that make AI relatable. For instance, explaining AI in manufacturing as a tireless assistant that predicts when machines need maintenance can make the concept more approachable. In logistics, AI can be likened to a smart driver who calculates the most efficient delivery routes. In healthcare, AI functions like a super-organized nurse, handling paperwork quickly so that staff can focus on patient care.
Framing AI as a helper rather than a threat can change perceptions. Instead of describing AI’s technicalities, emphasize its practical benefits. For example, instead of saying, “We’re using machine learning to optimize operations,” say, “This tool will help us reduce errors and speed up processes by 30%.”
Building Your AI Playbook
The first step in adopting AI is identifying problems, not technologies. Start by asking key questions: Where are we losing time or money? What tasks frustrate employees the most? Where do inefficiencies or errors frequently occur? Examples of pain points might include unplanned downtime in manufacturing, inefficient delivery routes in logistics, or administrative overload in healthcare. Once these problems are identified, businesses can look for AI tools tailored to solving them.
Starting small and scalable is essential. Pilot projects allow companies to test AI solutions with minimal risk. For instance, manufacturers can use predictive maintenance tools like UpKeep to monitor equipment health, while logistics companies can optimize delivery routes using platforms like Route4Me. Data visualization tools such as Tableau or Microsoft Power BI can help businesses analyze performance metrics easily.
Leveraging existing data is another practical step. Many companies already have valuable data from sources like machine sensors, GPS tracking, or patient records but don’t realize its potential. Analyzing this data with simple tools like Excel or Power BI can lay the foundation for more advanced AI applications.
Inspiring Real-Life Success Stories
AI’s potential is best illustrated through real-world examples. Siemens implemented AI for predictive maintenance, reducing factory downtime by 20% and saving significant costs. Similarly, Maersk used AI to optimize cargo loads and shipping routes, improving efficiency and lowering emissions. In healthcare, Cleveland Clinic applied AI to predict patient admissions and optimize staffing, which resulted in shorter wait times and better care delivery.
Â
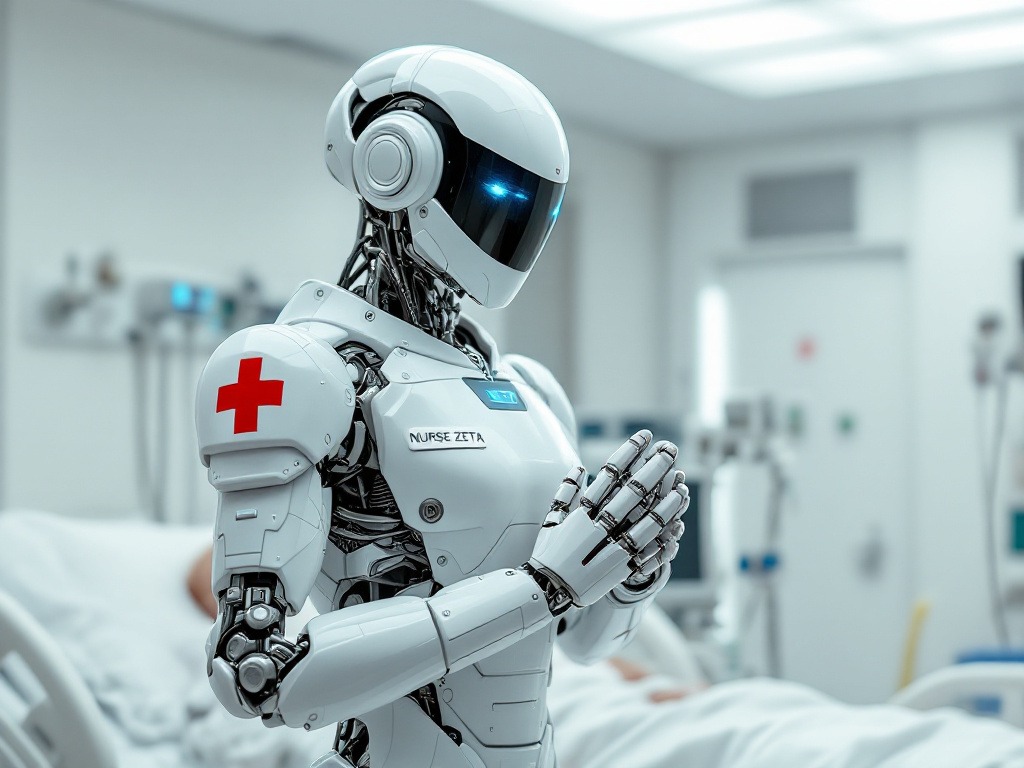
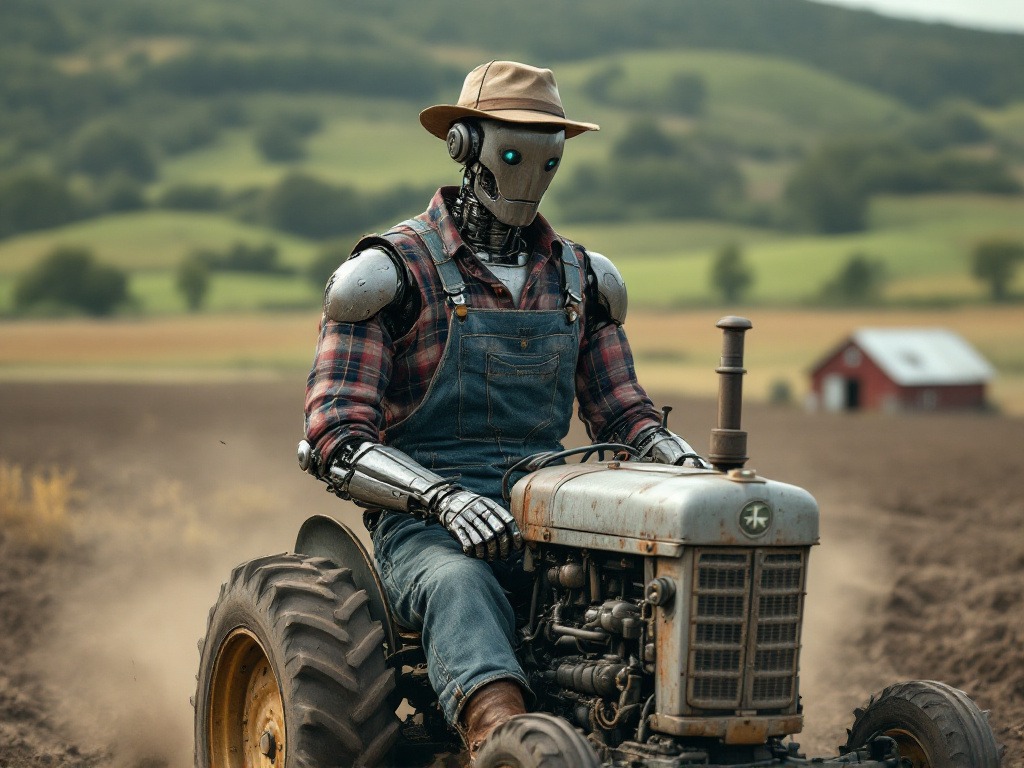
Overcoming Challenges in AI Adoption
Employee resistance can hinder AI initiatives. To overcome this, involve employees early in the process and show how AI will make their work easier, such as automating repetitive tasks. Providing training opportunities further ensures a smooth transition. Platforms like LinkedIn Learning and DataCamp offer courses specifically designed to upskill teams in using AI tools effectively.
Upskilling is critical for long-term success. Training employees to understand and utilize AI not only empowers them, but also reduces reliance on external consultants. This builds a culture of innovation and adaptability within the organization.
Final Takeaways
Adopting AI doesn’t have to be daunting. Begin with small, manageable projects that address specific pain points. Use relatable language to communicate the benefits clearly and build confidence by leveraging existing data. AI is not magic—it’s a tool that, when used thoughtfully, can transform traditional industries.
By embracing these steps, companies can bridge the gap between AI’s complexity and their operational needs. Start today, and unlock the potential for innovation and efficiency in your industry.